WordPress generally works fairly well on small-to-medium sites; on larger sites, it can run into performance issues because of the size of the database.
A current project I’m working on for LuminFire has a WooCommerce store with potentially 270,000+ customers, and that’s causing some issues with site performance. For sake of development, our dev store has 500,000+ users.
Contents:
Generating Dummy Users
First, I generated a CSV file with 50,000 fake users using mockaroo.com and imported them using this plugin (I had to bump up my PHP memory limit to 4096MB and execution time to 240 and it still timed out a couple of times, but I deleted the users who had already been imported and then ran the import again).
Update: I have since learned about WC Smooth Generator, and it may have worked just as well or better.
I figured 50K unique users were plenty and duplicating them via MySQL queries was more efficient, so wrote these queries to do the job.
The users
queries ran in seconds each, copying a batch of 50K rows at a time. The usermeta
queries, not so much…they took about 11 minutes each since there were about 1.71 million rows to clone each time.
In case it’s helpful to you, here are files with the dummy users:
- CSV with 50K users
- MySQL dump of
wp_users
table (30.4MB)
- It does include the ID field since it needs to match the
usermeta
table.
- The first user ID is 510024; if you try to import and have user IDs above 510024, you’ll have errors.
- 500,000 rows
- MySQL dump of
wp_usermeta
table (148.5MB)
- It does not include the
meta_id
field (so no errors trying to overwrite existing IDs).
- 17,000,000+ rows
WordPress User Dashboard
At the top of the user dashboard, WordPress typically displays a list of the user roles on your site, as well as the number of users in each role.

The number of each users is generated by the count_users
function, which uses a resource-intensive SQL query. In our dev site, it takes 15+ seconds just to run the query.
This is a known issue and should be resolved in WordPress 5.0 (currently scheduled for release in late 2018), but we need this working much sooner.
WordPress Multisite drops the number of users per role and instead shows just a list of roles; that’s how WordPress.com and other large multisite networks avoid this performance hit.
The proposed patch on the ticket modifies the count_users
function to behave similarly to WP Multisite: if there are more than 10,000 users (modifiable with the new wp_is_large_user_count
filter), it doesn’t show the number of users per role.
Since the patch is for the development version of the WP code, it doesn’t apply cleanly to a production site, so I manually patched WP core. Here are several patch files for different versions of WordPress; make sure to use the appropriate patch for your version:
Core Patch File
You can apply this patch file by downloading it, opening your WordPress installation in a terminal, and running git apply <path-to-downloaded-patch-file>
.
WooCommerce User Queries
The biggest performance hit I found was searching for customers when editing an order; it took 10–15 seconds for search results to be returned.
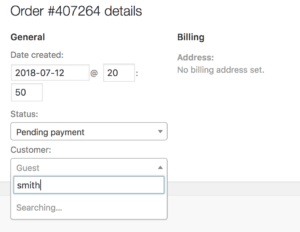
- If searching by customer ID, the backend would respond pretty quickly.
- Otherwise, it runs a full text search for the search term in the first and last name, which takes a while; see the code for full details.
Since orders aren’t manually assigned/reassigned too often, we decided this behavior was acceptable for now.
WooCommerce Customer Reports
The WooCommerce customer reports were the other major performance hit. For now, we simply disabled the customers reports since this particular customer already has a third-party system where they manage the customer data, so they’re not likely to use the WooCommerce reports.
Customers vs. Guests
Timing details:
- Around 20–40 sec to load the page
- Around 7 sec to get administrator users
- Around 7 sec to get shop_manager users
- Around 6 sec to get all other users
Here are the actual actual MySQL queries. In my staging environment, PHP ran out of memory; in my local environment, it took 305MB(!) to load the report for 1 week (the default view).
As noted above, we gave up on optimizing this report since the customer doesn’t really need it.
Customers List
This was completely unusable; it took 300 seconds to load the page.
Here are the actual MySQL queries. Two JOIN
s times in each query with full-text search on two columns × three queries was just too much on such a large table.
As noted above, we gave up on optimizing this report since the customer doesn’t really need it.
WordPress Posts Dashboard
Update: found another place where there’s a 15+ second wait. When bulk-editing posts (or any other post type that supports authors), an author dropdown causes a full search of the wp_usermeta
table. I’ve updated the patch file in the gist above to include a fix for this.
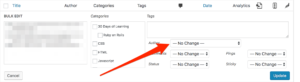
Summary
In summary, having 500,000 users on a WordPress and WooCommerce site doesn’t hurt overall performance too much, as long as you include an upcoming change in WP core and can get by without the WooCommerce customer reports.
We may explore further options to improve performance particularly when getting user roles from wp_usermeta
, and I will update this post or add a new post if we find other enhancements.